Self-driving cars (aka driverless cars or autonomous vehicles) are among the most visible faces of Artificial Intelligence (AI) today. In continuation with Shreeharsh Kelkar’s excellent post on Artificial Intelligence last month, I would like to pick up his lead and complicate yet another story of AI – the story of the relationship between software developers and machine learning algorithms. For this purpose, I will use my ongoing field work among members of an academic research group as an example. The work of this particular research group centers around an experimental vehicle – a self-driving car (hereafter: SDC).
During my field work I experienced a couple of surprises which challenged my earlier assumptions about the research on SDCs. That is, I previously assumed that the many promises invested in machine learning would lead to its extensive use in the experimental vehicles. However, this is not the case. I started to wonder why the researchers refrain from using machine learning even though they are officially members of an AI department. After introducing you to the field of SDC research in this first part, I will provide you with three tentative answers in an upcoming second post.
A Big Mess – Situating Research in Self-driving Cars
Whenever I try to situate research in SDCs, I am confronted by a big mess. If you follow its ties to the world of cars and driving, you end up with a versatile and pervasive artifact – the automobile – with so many layers of meanings and diverse communities of practices. With 1 billion of them existing, cars dominate transportation in many countries of the world. SDC research is tied to multiple contexts. Several origin stories circulate in the field. That is why, in this post I do not even try to map the different contexts in which cars and self-driving car research are situated. I also refrain from telling one of the origin stories of self-driving-cars, although I do recommend reading “Driving the Dream” by Jameson M. Wetmore. His history of Automatic Highway Systems can be read as a marginalized back-story to self-driving cars. Instead of doing all the above things, I want to introduce you to this field by briefly drawing your attention towards two more recent events which turned out to be highly influential among the members.
Major Event #1 – DARPA Grand Challenges
In 2000 U.S. Congress passed a mandate that by 2015 one-third of the operational ground combat vehicles of U.S. military are to be unmanned. In response to this, DARPA organized a series of “Grand Challenges” in 2004, 2005, and 2007. During these events robotics researchers competed with their autonomous vehicles for a cash prizes by solving tasks, likewise completing an autonomous drive through the desert. In this case autonomous means that the cars are unmanned and perform without remote control by the competing teams.
In SDC research this series of events is usually told in the form of a narrative of progress. During the first Grand Challenge (2004) no vehicle finished the race through the desert. The following year a handful of vehicles succeeded. By 2007 several teams completed a series of tasks in a mixed human and nonhuman scenario which simulated suburban traffic at an abandoned airbase. Among the members of the field it is widely assumed that this development – in only 3 years – provides a proof of concept. From their perspective mastering everyday traffic is not only feasible for SDCs but only a matter of time until the technology has fully matured.
and the winner is…
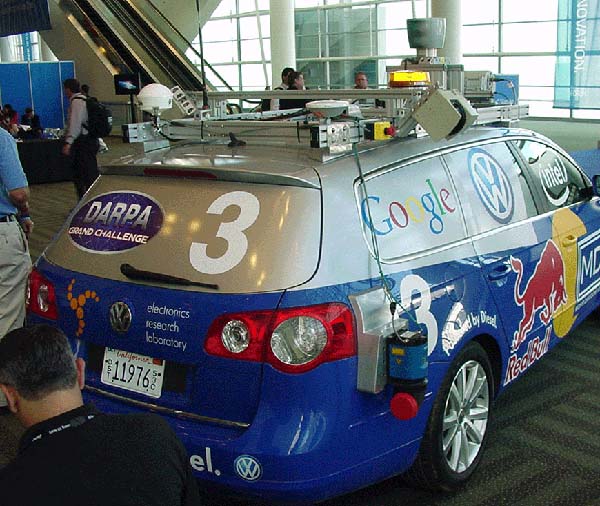
Stanford Vehicle for the DARPA challenge. Photo taken from Flickr. Licensed under a Creative Commons license.
Even if one doubts this techno-optimism, one thing is clear. DARPA succeeded in enrolling a large bunch of influential researchers and thus, enlarged a research community that has been marginal before the Grand Challenges. In addition, by creating these events it steered the research community in a specific direction. A direction that primarily serves the interest of the military applications of SDCs. Steven E. Shladover argues:
The needs of the civilian road transportation and military transportation communities, as identified above, are strikingly different from each other. […] When these differences are considered in toto, significant doubts arise about the extent to which the technologies and designs developed to meet the one set of needs will be transferable to the other domain. (2009:13)
Major Event #2 – Google’s Self-driving Car Project
In 2010 Google announced its SDC project. At the time of this writing Google operates the largest fleet of experimental vehicles in the world. However, this is not the sole reason why Google is a big player in the field. It can be argued that Google has made a major contribution to the normalization of SDC research. Until Google’s project very few teams conducted test-drives in real-life traffic. Google has not only tested its SDCs in over 300,000 miles of driving under everyday conditions but also successfully lobbied for new traffic laws in Nevada and California.
Due to its media attention the Google project has become a technological standard to which other SDC projects are measured up against. Let’s have a look at how Google performs its test-drives.
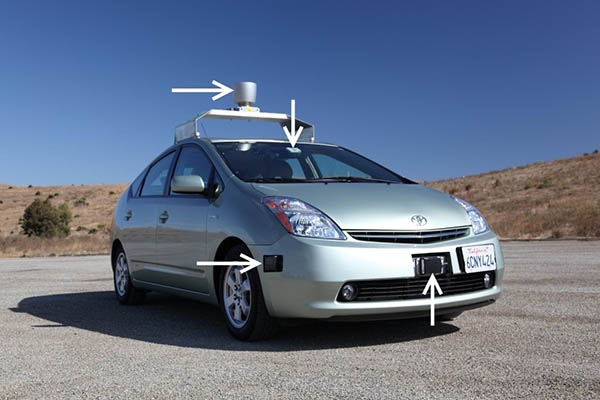
The Google Self-driving Car with its sensors. Original photo taken from Flickr and subsequently modified. Released under the CC license.
Above you see a picture of an experimental vehicle, in this case a modified Toyata Prius. The arrows point towards a variety of sensors: laser range finders on the roof, RADAR on the side, cameras behind the windshield. What you don’t see is an high-accuracy odometer and a differential GPS receiver.
How does it work?
In short, the SDC works like this. All the signals from the sensors are processed by computers to make sense of its surrounding environment and to localize itself on a digital high-precision map. On the basis of the sensor data and the position on the map, the SDC makes decision on what to do next. This is only a very brief description of what actually happens, but it will suffice for now. As the text in the box at the bottom of the picture explains, the SDC is never driving by itself in everyday traffic. It is closely monitored by a safety driver and an engineer while driving in public. The safety driver can resume full control of the car whenever s/he wishes. Whenever the SDC fails to master a given situation, the safety driver will disconnect the computers and resume driving manually.
I thought that autonomous driving research would incorporate a great deal of machine learning algorithms. But there I was surprised. In part two of my post, I will talk about the relationship between software developers and machine learning algorithms in the domain of SDC research.
2 Trackbacks