In the film Doctor Dolittle (1967), the title character yearns to “Talk to the Animals,” as the song goes, to understand their mysterious and often vexing ways. It is interesting to observe a similar impulse to understand and communicate with algorithms, given their current forms of implementation. Recent research shows that intense frustration often emerges from algorithmically driven processes that create hurtful identity characterizations. Our current technological landscape is thus frequently embroiled in “algorithmic dramas” (Zietz 2016), in which algorithms are seen and felt as powerful and influential, but inscrutable. Algorithms, or rather the complex processes that deploy them, are entities that we surely cannot “talk to,” although we might wish to admonish those who create or implement them in everyday life. A key dynamic of the “algorithmic drama” involves yearning to understand just how algorithms work given their impact on people. Yet, accessing the inner workings of algorithms is difficult for numerous reasons (Dourish 2016), including how to talk to, or even about, them.
Talking about “Algorithms”
Common shorthand terms such as “the algorithm” or even “algorithms” are problematic considering that commercial algorithms are usually complex entities. Dourish (2016) notes that algorithms are distinct from software programs that may make use of algorithms to accomplish tasks. The term “algorithm” may also refer to very different types of processes, such as those that incorporate machine learning, and they may be constantly changing. For the purposes of this discussion, the term “algorithm” will be used while keeping these caveats in mind. In this post, I relate the findings of ethnographic research projects incorporated into the new volume, The Routledge Companion to Media Anthropology (Costa et al. 2022), in which interviewees use the term to narrativize troubled interactions they experienced with devices and systems that incorporate algorithmic processes.
Simply pinpointing how algorithms work is a limited approach; it may not yield what we hope to find (Seaver 2022). In studying technologists who develop music recommendation algorithms, Nick Seaver (2022) reminds us that algorithms are certainly not the first domain that ethnographers have studied under conditions of “partial knowledge and pervasive semisecrecy” (16). He lists past examples such as magicians, Freemasons, and nuclear scientists. Seaver argues that researching algorithmic development is not really about exposing company secrets but rather more productively aims to reveal “a more generalizable appraisal of cultural understandings that obtain across multiple sites within an industry” (17). A different approach involves exploring algorithms’ entanglements in an “ongoing navigation of relationships” (15). The idea is to reveal the algorithmic “stories that help people deal with contradictions in social life that can never be fully resolved” (Mosco 2005; see also Zietz 2016).
Narrativizing Algorithmic Experience
In order for data to “speak” about a domain vis-à-vis a group of users, data must be narrativized (Dourish and Goméz Cruz 2018). In the domain of machine learning, large-scale data sets accumulated from the experiences of many users are subsequently used to train a system to accomplish certain tasks. The system then must translate the processed information back so that the information is applicable to the individual experiences of a single user. Algorithmic data must ultimately be “narrated,” especially for devices that have the potential to “re-narrate users’ identities in ways that they strongly [reject] but [cannot] ignore” (Dourish and Goméz Cruz 2018, 3). Dourish and Goméz Cruz argue that it is only through narrative that data sets can “speak,” thus extending their impact, albeit in ways that may differ significantly from designers’ initial conceptions.
In light of this context, responding to algorithms through ethnographic narrative emerged as an important theme in a volume that I had the pleasure of co-editing with Elisabetta Costa, Nell Haynes, and Jolynna Sinanan. We recently saw the publication of our ambitious collection, The Routledge Companion to Media Anthropology (Costa et al. 2022), which contains forty-one chapters covering ethnographic research in twenty-six countries. The volume contains numerous chapters and themes pertaining to science and technology studies, including a section specifically devoted to “Emerging Technologies.” Additional themes include older populations’ struggles with technology, Black media in gaming ecosystems, transgender experiences on social media, and many other relevant themes. The volume also collectively tackles numerous media types including digital spaces, virtual reality, augmented reality, and historical media forms.
Talking Back to the Algorithm
One science and technology-related theme that emerged across different sections of the volume involved perceptions of algorithmic usage and impacts on individuals. Several chapters explored identity characterizations that users of technologized devices, such as the Amazon Echo and the Amazon Echo Look, as well as spaces such as the video-sharing platform of YouTube, found violative of their sense of self and well being. In her chapter, “Algorithmic Violence in Everyday Life and the Role of Media Anthropology,” Veronica Barassi (2022) explored the impacts of algorithmic profiling on parents through an analysis of Alexa, the voice-activated virtual assistant in the home hub Amazon Echo. The chapter examines the experiences of families using Alexa in the United Kingdom and the United States during her study from 2016-2019.
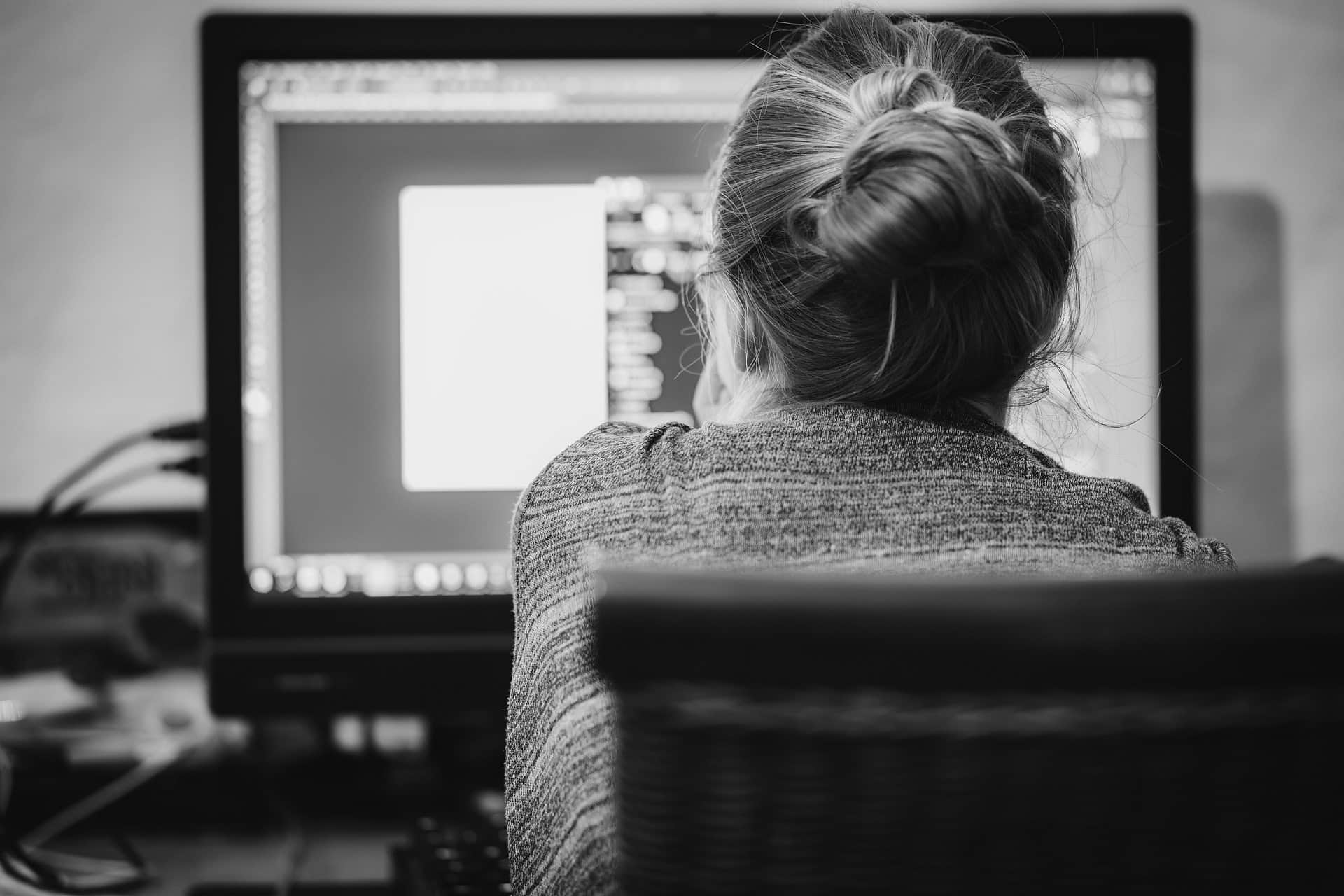
Talking back to the algorithm. Image from Pixabay.
Participants in Barassi’s study often felt that the product engaged in demeaning algorithmic profiling of them. For example, a woman named Cara whom Barassi met in West Los Angeles related how angry and irritated she felt because she was automatically targeted and profiled with weight loss ads simply because she was a woman over 50 years old. Feeling belittled by such profiling, she told Barassi, that “there is so much more to me as a person.” Amy, another participant in her study who was actively trying to lose weight, felt that algorithmic profiling concentrated on a person’s vulnerabilities, such that every time she went on Facebook she was bombarded with ads for new diets and plus-size clothing. She too used exactly the same phrase, that there was “so much more to her as a person,” than the algorithmically-generated profiles constructed.
The commentary that Barassi collected from participants represents an attempt to “talk back” to the “algorithm” or perhaps more accurately the developers, companies, and societal perspectives that have collectively implemented violative profiling. By relating their experiences, these narratives work to counteract the feelings of powerlessness that many interviewees felt in the technologized construction and representation of their perceived identity.
Another important aspect of Barassi’s contribution is to broaden analysis of algorithmic creation and impact beyond a particular piece of technology, and understand how algorithmic profiling and predictive systems are deeply intertwined with forms of bureaucracy. She observes that algorithmic profiling engages in symbolic violence because it “pigeon-holes, stereotypes, and detaches people from their sense of humanity” (Barassi 2022, 489). Barassi argues that we must attend far more closely to the relationship between bureaucracy and structural violence as instantiated in algorithmic profiling.
Similar interviewees’ experiences of feeling belittled by algorithms emerged in the chapter by Heather Horst and Sheba Mohammid, entitled “The Algorithmic Silhouette: New Technologies and the Fashionable Body” (2022). Horst and Mohammid studied the Amazon Echo Look, an app that compares outfits and provides fashion recommendations based on expert and algorithmic information. Priced at $199 and released widely in the US market in 2018, it was ostensibly perceived as a democratizing fashion tool, given that the average user would not ordinarily have daily customized access to fashion expertise.
Horst and Mohammid examined use of the device among women in Trinidad in 2019-2020. One of its features was called Style Check, in which the user selected and tried on two different outfits and submitted images of themselves wearing the outfits for the device to compare. It provided a percentage preference ranking, along with a narrative about the recommendation. Women in the study noted that the system could be very useful for providing recommendations and affirming their choices, particularly for meeting their criteria to appear professional in their wardrobe.
Yet some women felt that the device misrecognized them or their goals in oppressively normative ways. In one instance, a woman was threatened to be banned from adding images due to violating “community guidelines” because she was trying to compare herself wearing two bikinis. Another woman complained that the device’s recommendations seemed to be geared to select garments that made her appear slimmer. In an interview she noted:
It’s assuming that you want a slimmer silhouette, less curves, less flare…it doesn’t take into consideration me, like my personal preferences. It’s comparing me basically using its algorithm and how do I know that your algorithm is as inclusive as it should be, you know?
They conclude that these tensions reveal complexities that emerge when devices do not translate across cultural contexts. Their research demonstrates how inherent biases as instantiated in devices and systems reproduce structural inequality. Horst and Mohammid (2022) recommend analyses that can “give feedback to designers and others at particular points in the life of algorithms and automation processes” (527). They recommend taking a “social life of algorithms” approach that considers how algorithmic processes are embedded in cultural and social relations, and how particular values become normative. Feedback from people interacting with algorithmic products needs to be collected and circulated, particularly to challenge the “inevitability” narrative of technical impact that often accompanies the emergence of new technologies.
Zoë Glatt (2022) writes about perceptions of algorithms among YouTube influencers in London and Los Angeles between 2017 and 2021 in her chapter, “Precarity, Discrimination and (In)Visibility: An Ethnography of ‘The Algorithm’ in the YouTuber Influencer Industry.” Drawing on fieldwork among hard-working videographers, video editors, performers, and marketers, Glatt’s chapter traces how people respond to algorithmic recommendations on the YouTube platform, which directly impact influencers’ livelihoods. She found that “algorithmic invisibility,” or having work deprioritized or omitted on recommendation lists based on algorithmic rankings, is a common fear even among successful content creators with sizable followings. One vlogger expressed her deep concerns about platform invisibility:
Over the past year it has all gone to hell. There’s just no pattern to what is happening in essentially my business, and it is scary and it’s frustrating. I don’t know if people see my videos, I don’t know how people see my videos, I don’t know what channels are being promoted, I don’t know why some channels are being promoted more than others. There’s just no answers, and that’s scary to me. (Excerpted from a vlog by Lilly Singh 2017)
Glatt makes the important contribution of analyzing the cultural and economic meanings that creators attach to assumptions about algorithms. In triangulating what creators say about algorithms with how they feel about them, and the actions that influencers take in response, Glatt provides an important framework for parsing algorithmic interactions in culture. Glatt’s findings that influencers found the algorithm to be unpredictable and stressful underscore the importance of researchers to help hold developers and implementers accountable for algorithmic processes, particularly with regard to addressing the algorithmic discrimination that participants reported.
Collectively, these chapters in the Routledge Companion to Media Anthropology include crucial analysis about ethnographic interviewees’ perceptions of algorithms while also providing a mechanism for participants to “talk back” to “algorithms” about how they as individuals are represented in everyday life through technology. Indeed, the stories presented serve as a reminder that it is important to think of algorithms relationally and to provide consistent mechanisms for feedback and implementation strategies to reduce harm. It is indeed time to “talk to the algorithms” by engaging users as well as the designers, processes, and societal organizations that implement them in daily life. We can move on from pinpointing exactly how algorithms work, to shifting attention to establishing ways to meaningfully incorporate feedback to change their impact on human beings around the world.
References
Barassi, Veronica. 2022. “Algorithmic Violence in Everyday Life and the Role of Media Anthropology.” In The Routledge Companion to Media Anthropology. Edited by Elisabetta Costa, Patricia G. Lange, Nell Haynes, and Jolynna Sinanan, 481-491. London: Routledge.
Costa, Elisabetta, Patricia G. Lange, Nell Haynes, and Jolynna Sinanan. 2022. The Routledge Companion to Media Anthropology. London: Routledge.
Dourish, Paul. 2016. “Algorithms and their Others: Algorithmic Culture in Context.” Big Data & Society (July – December): 1-11.
Glatt, Zoe. 2022. “Precarity, Discrimination and (In)Visibility: An Ethnography of ‘The Algorithm’ in the YouTube Influencer Industry.” In The Routledge Companion to Media Anthropology. Edited by Elisabetta Costa, Patricia G. Lange, Nell Haynes, and Jolynna Sinanan, 544-556. London: Routledge.
Horst, Heather A. and Sheba Mohammid. 2022. “The Algorithmic Silhouette: New Technologies and the Fashionable Body.” In The Routledge Companion to Media Anthropology. Edited by Elisabetta Costa, Patricia G. Lange, Nell Haynes, and Jolynna Sinanan, 519-531. London: Routledge.
Mosco, Vincent. 2005. The Digital Sublime: Myth, Power, and Cyberspace. Cambridge, MA: The MIT Press.
Seaver, Nick. 2022. Computing Taste: Algorithms and the Makers of Music Recommendation. Chicago: The University of Chicago Press.
Ziewitz, Malte. 2016. “Governing Algorithms: Myths, Mess, and Methods.” Science, Technology, & Human Values 41(1): 3-16.