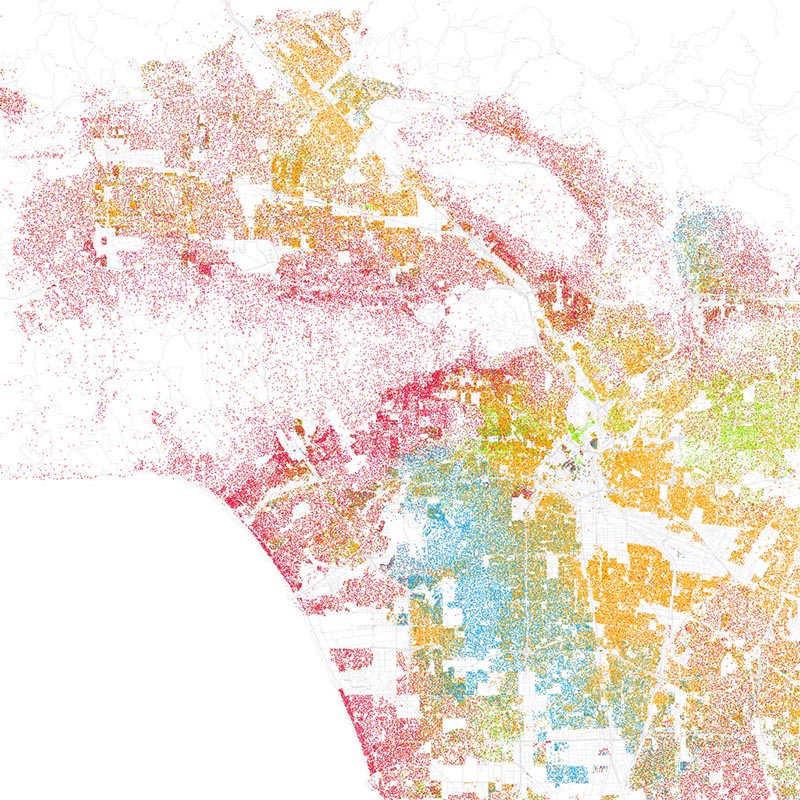
Data visualization of Los Angeles by race and ethnicity. By Eric Fisher via Flickr, CC BY-SA 2.0.
In early November 2016, ProPublica broke the story that Facebook’s advertising system could be used to exclude segments of its users from seeing specific ads. Advertisers could “microtarget” ad audiences based on almost 50,000 different labels that Facebook places on site users. These categories include labels connected to “Ethnic Affinities” as well as user interests and backgrounds. Facebook’s categorization of its users is based on the significant (to say the least) amount of data it collects and then allows marketers and advertisers to use. The capability of the ad system evoked a question about possible discriminatory advertising practices. Of particular concern in ProPublica’s investigation was the ability of advertisers to exclude potential ad viewers by race, gender, or other identifier, as directly prohibited by US federal anti-discrimination laws like the Civil Rights Act and the Fair Housing Act. States also have laws prohibiting specific kinds of discrimination based on the audience for advertisements. In its response to the Propublica investigation, Facebook claimed that its policies prohibit third party use of data and its system for discrimination. But if data were not collected for discrimination, why collect at all? When a site like Facebook, or any of the many other tech companies, collect and categorize data it is not done solely to hold the data in storage. Collecting and categorizing the data is about making distinctions between different things. In other words, discrimination is inherent in big data systems. It should not, then, be surprising when that discrimination brings about a negative outcome.
Data discrimination in the wild
The threat of data-based discrimination is not exclusive to Facebook. And the use of data emanating from users of online spaces including social media, shopping, and travel services for discriminatory purposes is not a new phenomenon. In 2012 the Wall Street Journal reported that the travel site Orbitz was showing visitors using Macs different hotel rooms than those shown to PC users. Orbitz’s decision to show users different hotels was based on data that showed Mac users were more likely to book more expensive rooms than PC users. And the kind of technology you use is not, of course, the only personal marker ripe for making discriminatory decisions. Dr. Latanya Sweeney, director of Harvard University’s Data Privacy Lab, has found that searches for racially associated names were more likely to return Google AdSense ads with language suggesting an arrest. The ramifications of this being that organizations conducting searches for potential employees could be negatively influenced by the ad search results that may not actually apply to the job candidate.
Algorithmic decision-making—the use of a set of parameters to make choices—is de rigueur for many organizations. Algorithms using data like that collected by Facebook and Orbitz can perform many of the functions that may have taken humans significantly more time and/or effort to complete. According to Professor Nick Diakopoulos this includes prioritizing tasks, classifying info, identifying relationships, and filtering. Though efficient with these tasks, there has recently been significant attention given to detrimental possibilities of algorithmic decision-making. This past May, the White House published a report on big data, algorithms and civil rights. The Open Technology Institute similarly published a collection of essays examining the possibilities of data and discrimination. And of course there have been academic and professional conferences and symposia considering the possible negative effects of big data.
Artifacts and politics
The tenor of news reports, some academic literature, and statements from some professionals is general surprise that algorithms and the data they use could result in bias or be used to perpetuate negative outcomes. The rhetoric surrounding big data and algorithms point to systems that were to provide insights into humanity and organizations in ways previously impossible. Big data could help identify flu outbreaks (not really), assist government agencies, and solve crimes. Big data and algorithmic systems are supposed to be really smart, all the while being dumb. That is, the machine was not to have the same susceptibility to bias and discrimination as its maker.
In a well-known work, Professor Langdon Winner asks, “Do Artifacts Have Politics?” Another way to frame this question is to ask whether you can create a system, process, product, a “thing” that is devoid of your own worldview, morals, values, etc. The answer is, decidedly, “no.” In his article, Winner describes his theory of technological politics, which requires attention to the meanings behind the characteristics of technology. The theory considers the power dynamics reflected in technological systems. Winner uses the examples of the heights of parkway bridges in Long Island to illustrate how mundane technology (broadly defined) could be equipped with the ideology of its creator. He goes further to demonstrate, using the implementation of the tomato harvester in California farmland, how technological developments can produce both breakthroughs and setbacks. Important is that, unlike the height of parkway bridges, which was said to have been set low enough to keep public transportation, and therefore poor people and people of color from the beaches, the implementation of the harvester was not an intentional use of technology for bias. Instead, according to Winner, the use of the harvester, which was a boon to those who could afford it, demonstrates “an ongoing social process in which scientific knowledge, technological invention, and corporate profit reinforce each other in deeply entrenched patterns that bear the unmistakable stamp of political and economic power.”
W(h)ither algorithms and big data
The system of big data and algorithms demonstrate both kinds of power dynamics Winner uses to illustrate the theory of technological politics. I would argue that big data is analogous to the tomato harvester—a seemingly neutral technology, the use of which reinforces social order through categories, labels, and classification. Algorithms, then, are like the parkway bridges—choices shaped by their creators’ perspectives, the outcomes of which could perpetuate power structures to negative effects. Should we reject the technology that, for some, offers so much hope for exploration and investigation, and the potential for understanding relationships in society at a scale and in a manner not before possible? That’s probably not going to happen. We can’t be surprised, however, when tech is used in a manner that reflects us. We should, however, take great care to lessen bias and consider power dynamics inherent and resulting from technology at its inception. The start of this, perhaps, is dispensing with the myth of neutrality in technology.
We are the problem. Yes, the medium is the message. That is, data, algorithms and other technology and the possible uses, reflect us, our biases, and our issues. They are but extensions of ourselves. This includes the potential to discriminate.
References and further reading:
Pasquale, F. (2015). The Black Box Society: The secret algorithms that control money and information. Harvard University Press.
Winner, Langdon. “Do artifacts have politics?.” Daedalus (1980): 121-136.