*A note from Co-PI Noopur Raval: The arrival and rise of gig-work globally has ushered in a new wave of conversations around the casualization of labor and the precarious nature of digitally-mediated “gigs,” ranging from online crowdwork gigs to digitally-mediated physical work such as Ubering. Gradually, scholarship has extended beyond North America and Europe to map the landscape of digital labor in the global south. These posts that make up “India’s Gig-Work Economy” are the result of one such project titled ‘Mapping Digital Labour in India,’ where four research fellows and a program manager, me, have been studying the dynamics of app-based ridehailing and food-delivery work in two Indian cities (Mumbai and New Delhi). This project is supported by the Azim Premji University’s Research Grants program. In this series of posts, the research fellows and I offer reflections on pleasure, surveillance, morality and other aspects woven into the sociality of gig-work and consumption in India. Each post also has an accompanying audio piece in an Indian language, in a bid to reach out to non-academic and non-English speaking audiences. The series ends with a roundtable discussion post on the challenges, gender and class dynamics, and ethics of researching gig-work(ers) in India.*
Download a transcript of the audio in Devanagari.
Ride-hailing[1] platforms such as Olacabs and Uber have “disrupted” public transport in India since their arrival. It has been almost seven years since app-based ride-hailing became a permanent feature of urban and peri-urban India with these aggregators operating in over a 100 Indian cities now. Akin to the global story, much has happened – there was a period of boom and novelty for passengers and drivers, then incentives fell. Ride-hailing work has become increasingly demanding with reduced payouts. But what hasn’t received enough attention (especially outside the US) is how these platforms create a deliberate regime of information invisibility and control to keep the drivers constantly on their toes which works to the companies’ advantage. What then are the implications of this uncertainty, which is fueled by app design as well as by the companies’ decision that drivers need little or no information about users? How does service delivery operate in a context where those actually delivering it have little or no idea about the workings of the system?
When algorithms make us not know
Algorithmic interactions form the core of the technology in ride-hailing apps through which service seekers and providers interact. As Lee et al. (2015) describe, “Algorithmic management allows companies to oversee myriads of workers in an optimized manner at a large scale, but its impact on human workers and work practices has been largely unexplored… Algorithmic management is one of the core innovations that enables these (cab-riding) services.”
Algorithms are procedural logics that produce different effects depending on the data they receive and the outputs they are optimized for (Wilson, 2016). Moreover, platform companies are not transparent about how their business logics contribute to these “optimizations”, which makes it difficult for all the stakeholders (passengers, drivers, police personnel, etc.) to make an accurate assessment of their functioning. This essay, then, explores how the lack of transparency around algorithmic structures not only prohibits drivers from knowing completely and surely about their work (“why did I get this ride?”, “why did my ratings drop?”) but also how they build tactics of coping and earning from a place of unknowing. Algorithms act as a regulator of work and their inherent structure constrains drivers from knowing fully about their work. Unknowing thus has two aspects: first, drivers do not have access or means to gather information; second, it is difficult to be sure of the existence of the said information in the first place.
In my research on ridehailing in the Delhi-National Capital Region (NCR), there were three things that I asked drivers about which led to ambiguous and inconsistent replies: how rides were allocated, how fares were determined and how ratings worked. While some drivers told me upfront they did not know how these systems worked, others offered explanations that they had devised or heard from somewhere else. For instance, not knowing what they will make per trip means that drivers plan their day in terms of target earnings instead of number of trips. Nearly all drivers I spoke to said they aimed to make Rs 1500-2000 (approx USD 20-25) per day in order to break even, irrespective of whether that goal requires 10 or 15 trips in a day. Yet not knowing what the next trip will earn them means they can’t refuse rides easily. Many drivers expressed discomfort about this fact, especially when compared to other means such as auto-rickshaws and traditional cabs where drop destination is known beforehand and fares can also be pre-negotiated, Unlike ride-hailing drivers, auto rickshaw drivers have the right to refuse passengers.
Many drivers now call passengers after accepting their booking to find out the destination. According to some drivers, this call also helped them understand the kind of passengers they were about to get and sometimes even allowed re-negotiation of the drop location to a mutually convenient spot if it was originally in a congested area. They also felt that assessing passengers before a trip was important so that they could act as mediators in the information gatekeeping process, because the passengers would have seen the fare already. For a driver, the lack of information added many layers of constant negotiation in a single trip—starting from the call to find out the destination to conversations during the trip to gauge potential earnings to finally suggesting alternative drop locations if there are any constraints in accessing the original destination—before they can claim their rightful earnings.
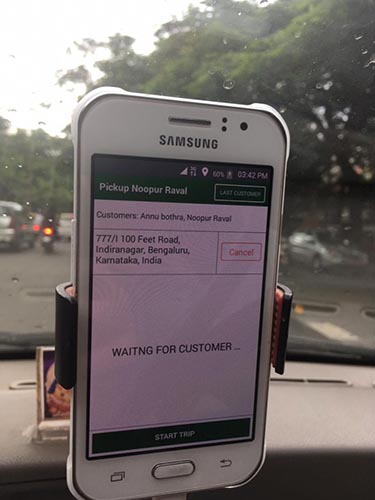
Ridehailing drivers only get the user’s name and pickup location as details about an upcoming trip. Photo by Noopur Raval
Knowing the terms of work—such as when work ends and begins, how the good jobs are being allocated and to whom, and an explanation of one’s income—is a foundation of formal and informal work. Such information is crucial because it allows us to separate our work and personal lives. Knowledge of these obviously quantifiable parameters can help drivers plan their earnings and investments and, crucially, when they can take a break based on much more or less work they have to do in order to meet their income targets.
Furthermore, as drivers showed me, ride-hailing companies spontaneously change the revenue model for “driver-partners” (as they are called) by sending them an SMS right before the change happens, thereby altering trip and mileage targets frequently to keep a degree of unknowability in drivers’ work. This unknowability disincentivizes drivers from going off the road as per their will and helps maintain a steady supply of cabs on the road. As Alex Rosenblat has demonstrated in her study of US Uber and Lyft drivers, they are compelled to accept rides without knowing their profitability. While the app design gives them an option to “choose” to accept or reject a ride, drivers are constrained by lack of adequate information pertaining to the trip as well as the rider in making this choice. The ‘information asymmetry’, as Rosenblat calls it, also feeds into drivers’ mistrust of the companies and their policies (Rosenblat, 2018). Moreover, these feelings and the uncertainty fed by unknowing were not limited to drivers. Passengers also noticed that a ride between two points could cost different prices at different times of day and they were not sure why or how this cost was calculated.
Unknowability as a form of knowing: A pedagogy of coping
As I observed in my interactions with drivers online and offline, new drivers often struggled with the degree of uncertainty and unknowability while more experienced drivers had accepted ‘not knowing’ and the opacity of the system as features of their work.
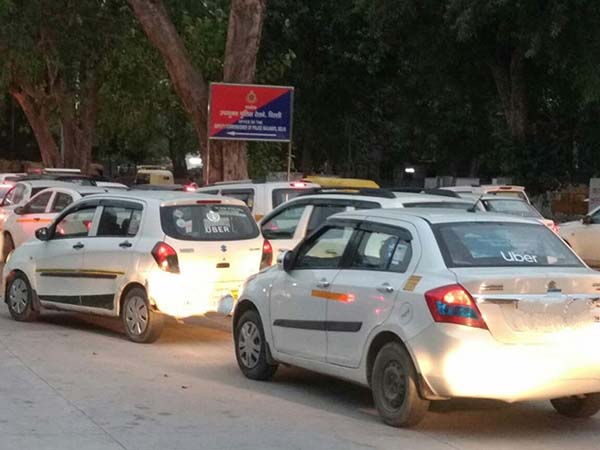
Not knowing enough about how much will a ride earn them means drivers are forced to be on the roads, often without a break. Photo by author.
Similar to what Rosenblat, Gray et al. and others have observed in the US, in India drivers were constantly engaged in meaning-making through communicative labor, i.e., sharing their experiences with other local drivers online and offline. Agreeing, reassuring, and repeating that drivers actually do not know enough through these discussions also gave them shared confidence in their own abilities and how they were approaching work despite being firmly rooted in unknowing. For instance, when I asked one Uber driver about how ratings worked, they said that all 5-star drivers were matched with 5-star passengers. Another Uber driver said that the higher a passenger’s ratings, the less time they would have to wait for pick-up.
Other forms in which this kind of unknowing manifested was the lack of a fare chart or any minimum or uniform rating system, leaving drivers to offer their own interpretations and coping strategies. For instance, a driver pointed out how very few rides are likely to be available in a specific suburb during hot afternoons and therefore he avoided dropping passengers to that location after 2PM.
How, then, does one learn to cope with such unknowable systems as a worker? And what values does such a pedagogy of coping with algorithmic opacity imbibe? In my fieldwork, apart from answering my questions, drivers were extremely interested in talking about the companies, including news about companies’ stock value, their futures, profits, etc. A persistent rumour in the field was that Reliance, the country’s largest telecom provider, was soon coming up with a competitor ride-hailing app, suggesting that there could be an incentive boom again. In online Facebook groups, drivers often discussed company CEOs’ salaries, comparing them to their own. On the flipside, when videos of ride-hailing and food-delivery drivers getting beaten up or arrested or cheated surfaced, drivers would comment with advice on how to safeguard oneself, how to deal with errant customers and so on. I interpret these practices of making sense of long and short-term work, framed as responses to constant ambiguity and uncertainty, as the development of an “algorithmic gut”.
This gut responds to the anxieties produced by platform infrastructure through a keen awareness of the shifts, the tweaks, the changes and the errors. And it orients how drivers approach and cope with their work by acknowledging that there is a lot unknown (and unknowable) in this kind of daily work. It also guides how drivers focus on the short-term (daily) goal of making profit, such as by tuning into peer groups both online and offline where grievances are discussed, collective action planned, and floating rumours assessed. This gut is an affective, sensorial attunement to how platforms are allocating and shifting power among drivers and plays a generative role in guiding drivers’ work decisions.
Conclusion
Uncertainty is an embedded part of a ride-hailing cab’s model of service delivery. For ride-hailing drivers, this ambiguity translates into less control over everyday negotiation of work as well as planning of financial assets for the future.
In my interactions, I discovered that drivers are certain that they will never know more than the company. What this has led to is a driver who is cynical but not entirely pessimistic. Drivers acknowledge that while companies and their structures may be problematic, what will keep them employed is passengers’ appetite for a service like this. They would like to imagine the future of their work but are cognizant of the dual challenge of the present: making money while struggling for self-preservation in order to perform immediate activities. Drivers are cognizant of an ambiguous future and even hesitant to engage in long-term planning. For now, they would prefer better earnings and greater control over how they perform labour. Hence, their focus is on devising specific strategies for known, short-term challenges instead of running after an unknown future.
References
Davis, Jenny L. 2014. “Triangulating the Self: Identity Processes in a Connected Era.” Symbolic Interaction 37 (4): 500-523.
Dodge, Martin and Kitchin, Rob. 2005. “Codes of life: identification codes and the machine-readable world.” Environment and Planning D: Society and Space 2005 (23): 851-881
Gray, Mary L., et al. 2016. “The Crowd is a Collaborative Network.” Proceedings of the 19th ACM conference on computer-supported cooperative work & social computing. ACM, 2016.
Kitchin, Rob. 2017. “Thinking critically about and researching algorithms.” Information, Communication & Society 20 (1): 14-29.
Lee, Min Kyung, et al. 2015. “Working with Machines: The Impact of Algorithmic and Data-Driven Management on Human Workers.” Proceedings of the 33rd Annual ACM Conference on Human Factors in Computing Systems.
Rosenblat, Alex & Stark, Luke. 2016. “Algorithmic Labor and Information Asymmetries: A Case Study of Uber’s Drivers.” International Journal of Communication 10: 3758–3784.
Ruckenstein, Minna and Mika Pantzar. 2017. “Beyond the Quantified Self: Thematic exploration of a dataistic paradigm.” New Media & Society 19(3): 401-418.
Willson, Michele. 2016. “Algorithms (and the) everyday”. Information, Communication & Society 10.1080/1369118X.2016.1200645
[1] Uber and homegrown Ola both started operations in India as ride-hailing services with the sharing options being added in 2015. Hence, the term ride-hailing has been used to describe these services which also includes ride sharing.
1 Comment
Nice content. It is very informative and I came to know about the situation of taxi drivers. It is obvious in India like the country they come from lower families and they have the burden of earnings so they don’t have any future plans for long terms. They think of short term plans. So, they have the right to know the algorithmic change of the companies they work in.
Also, I agree with this “new drivers often struggled with the degree of uncertainty and unknowability while more experienced drivers had accepted ‘not knowing’ and the opacity of the system as features of their work.”
Anyways, Thanks for sharing such information!