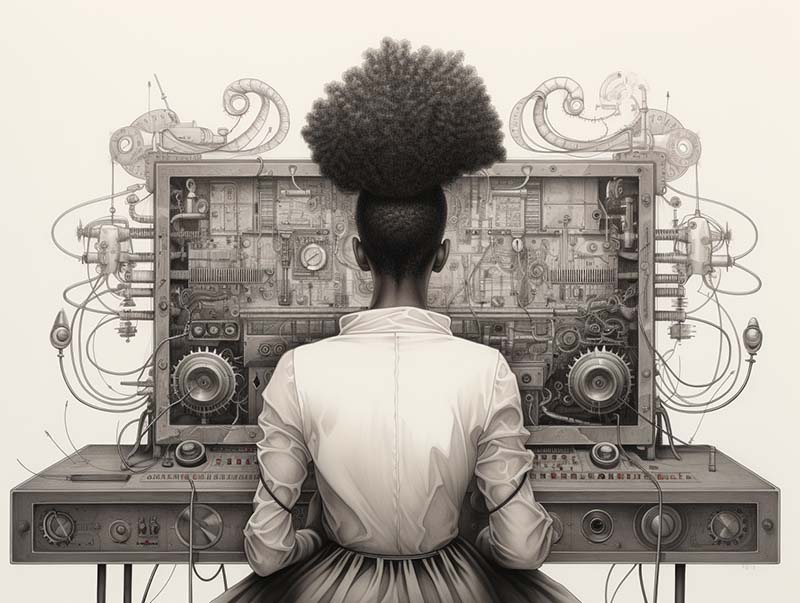
Scientist operating an algorithmic divination machine as imagined by Midjourney’s AI (image by Author & Midjourney).
Algorithms are tools of divination.[1] Like cowry shells, scapular bones, or spiders trapped under a pot, algorithms are marshaled to detect and relay invisible patterns; to bring to light a truth which is out there, but which cannot ordinarily be seen. At the outset, we imagine divination is a means to answer questions, whether in diagnosis of past events or for the prediction and guidance of future outcomes, choices or actions (Ascher 2002, 5). Yet, divination has an equally potent capacity to absorb the burdens of responsibility, to refigure accountability and, in so doing, to liberate certain paths of social action.
Algorithms are ideal technologies of mechanical divination[2] because they are already imbued with a rhetoric of “apersonality” (Bascom 1969; cited in Du Bois 1987, 101), as in, “the numbers don’t lie!” Like the caribou shoulder blades whose “cracks and burnt spots” are read by the diviner as a map to fertile hunting grounds, “the final decision about where to hunt… does not represent a purely personal choice” (Moore 1957, 70). Instead, it is diverted from the diviner and their client onto scapular burn marks and the ritual processes of their generation. Despite the diversity of divination practices, central to its rituals are those mechanisms designed to displace authorship and thereby legitimize the divinatory revelation as intrinsically detached and impartial. Algorithms, I Ching yarrow stalks, a poisoned fowl, even the chemical reactions of scientific experiments (DuBois 1987, 102; cf. Latour & Woolgar 1986), all allow a diviner to utter statements which they (seemingly) do not author (Boyer 2020 102; see also Silva 2014).
Such “ostensive detachment” (Boyer 2020), is often bolstered by the ritual deployment of aleatory mechanisms (cf. Argyrou 2002).
The specialist throws dice, or shuffles tarot cards, or empties a bag of small tokens on a table, processes that, from the standpoint of clients and other participants cannot be controlled. (Boyer 2020, 103)
Algorithmic divination, however, is never completely random. Casting dice may fall in “true” random fashion, but the divining done by algorithms is by design, codes and calculations, however complex these might be.[3] Like spirits who speak through the white noise of electronic static in EVP[4] (Espirito Santo 2013), algorithms manifest messages on a “chaotic blank canvas,” where chaos is a chaos of scale. Thus it is through an overabundance—an unmanageable, unwieldy, lumbering out-of-control elephant of data—that meaningful patterns are parsed. And just as cards must be turned over or laid out and dice must be thrown, movement is essential too to our digital divination (Espirito Santos 2013). It is in the logic of divination that algorithms move through or “process” data, extracting from the chaotic overabundance meaning through selection and, above all, connection.
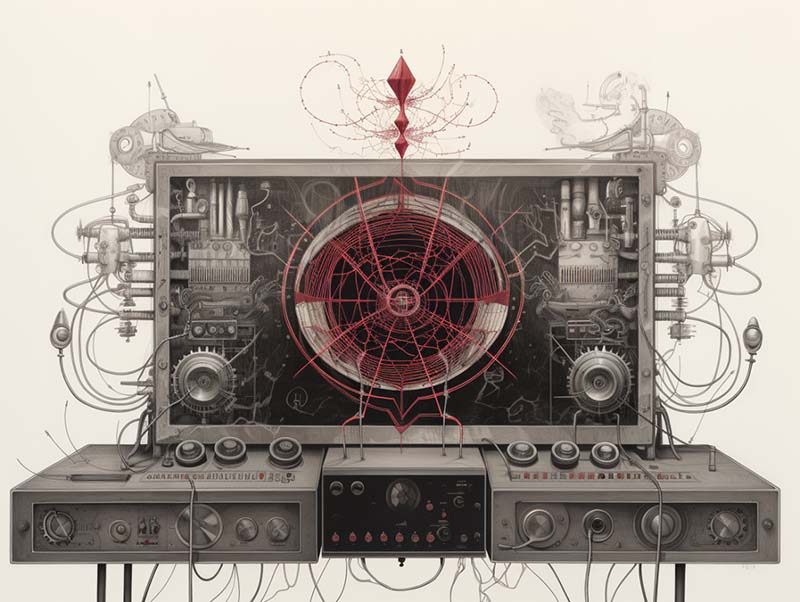
An algorithmic divination machine (image by Author & Midjourney).
Researchers argued early in the pandemic that Covid would be a “black swan event”; its course would be essentially unpredictable—“an unknown unknown” (Aven 2013, 45; cited in Khemasuwan and Colt 2020, 387). Into this uncertainty, researchers rushed to develop algorithmic approaches, particularly machine and deep learning models, which could be applied to various clinical and epidemiological problems presented by the virus. Describing their advantages for Covid-related research, Khemasuwan and Colt present what has become a routine understanding of AI and its applicability:
With the appropriate input and innovative algorithmic design, AI can recognise patterns, predict outcomes, assist with medical decision-making and help uncover relevant information from data. By seamlessly analysing millions of data points, AI is a potential game changer in the battle against the pandemic. (Khemasuwan and Colt 2020, 387)
Thus “millions of data points” are the staticky noise out of which algorithms effect divination, drawing statistically significant conclusions from otherwise unmanageable datasets. If formalized randomization is a mechanism for accessing unpredictable (and thus impartial) truths, then algorithms like AI learning achieve their own truth claims through the sheer scale of data.
Large language models (LLMs) are one example of algorithmic efforts to meaningfully harness an overabundance of information, too much for any one person to parse efficiently. Among these, OpenAI’s ChatGPT[5] presents perhaps the pinnacle of oracular detachment. Heikki found his team of programmers consulting with the LLM during a conference code jam in September 2023. The team of both professional software developers and amateur coders was tasked with porting code from one programming language (Python) to another (PHP). They found ChatGPT worked effectively alongside more established tools (e.g. Stack Overflow) when it came to checking some programming idiosyncrasies across the languages. ChatGPT played the role, in effect, of a senior colleague who could be consulted on demand. Of course, the advice given by any LLM is not always sound, but still, as with any correctly performed mechanical divination, ChatGPT never fails to give an “oracle’s pronouncement” with conviction absolute.
Of course whether it is the gods or mathematics, in the end, neither truly speaks itself. For now, “technical elites” continue to play the intermediary role of oracle or seer, interpreting the outcomes of machine learning (Larsson and Viktorelius 2022).[6] Deciding what counts as an input and interpreting what the output “means” is the work of a human, a political actor enmeshed in the local,[7] and really that actor is not just interpreting the answers but at the same time instantiating the questions.
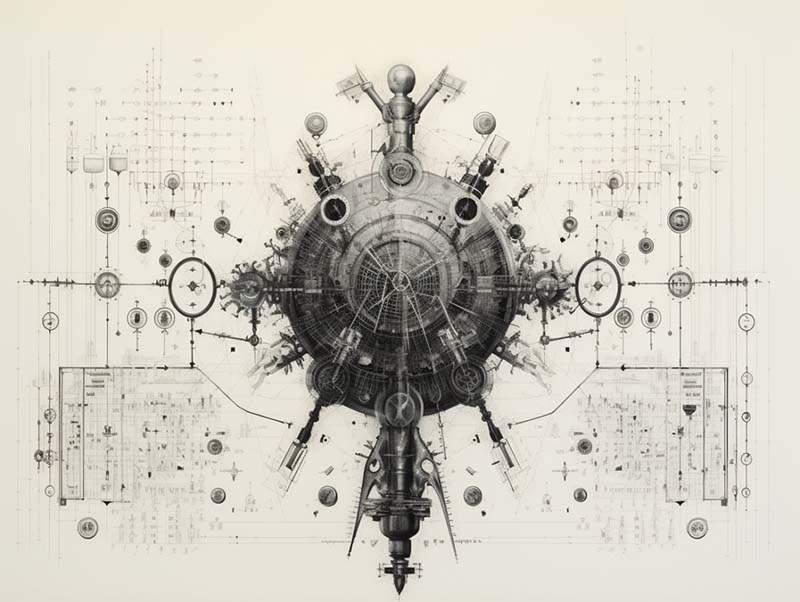
An algorithmic divinatory calendar (image by Author & Midjourney).
In Japan, as elsewhere, experimental research with machine learning is expanding alongside the accelerating accumulation and availability of “big data.” In bioscience, this includes caches of human biometric data, clinical case reports, and imaging; and other genetic, tissue, and blood samples, all collected and stored by hospitals, national biobanks, and consortiums. These medical repositories are the “superabundance” (Werbner 1976), the chaos of noise onto which algorithms are, in this case, ritually thrown. As scientific research questions become “mediated through predictive technologies” (Amelang and Bauer 2019, 496), Rebecca’s research has asked whether access to these datasets is transforming scientific decision making, where the mere availability of data is used like burnt scapula marks to map new research questions. In an inversion of idealized scientific practice, this is often directed by what algorithms can be used to divine rather than driven by pre-established hypotheses (Carlson 2022). Algorithmic work can even take on a kind of pre-divining procedure. Decisions about what research to conduct is distributed by scientists onto data’s potential, calling on it to determine in the first place what questions should be thereafter divined, just as diviners may ask preliminary details from clients, or consult about which questions should be asked, or what the best course of questioning might be to achieve a desired aim.
In one example, a bioinformatician at a Japanese national university described the way he sorted through publicly available chest x-ray data to mine it for research potential. Driven, as he explained, by the feeling that chest x-rays “contain a lot of information [that] is not used” since “cardiologists only check the heart and vessels and pulmonologists only checks the lungs,” he focused on a “reversal,” as he described it, asking what a deep learning model could predict from the x-rays themselves and their accompanying electronic patient data: disease diagnosis, age, sex. In a feedback loop, this allowed him to prepare his training data and his predictive model, based solely on the availability of these labels. In explanation, he stated simply: “One of the reasons to do such research is there is information” for it. He continued, “AI is black box itself… there are more than one hundred million parameters that predict [something] so we cannot see what they are thinking inside.” Even in the scientist’s expert “sociotechnical imagination” (Jasanoff and Kim 2015), the divinatory parallels are abundant. Algorithms are tools for revealing unknown patterns and information that can’t normally be accessed or “seen,” by way of inner workings that can’t (or won’t) be fully explained, with the revelations ultimately and by design credited to no one.
Of course, this obfuscation is not without consequence. In the ChatGPT example, for instance, OpenAI hasn’t disclosed the origin of all the training materials used to build their models. There is a clear conflict between open source advocates and AI model profiteers. On the one hand, the models’ success is based on the availability of huge open source code archives. On the other hand, the pay-walled developer features such as code completion in editing software is clearly against the spirit of the open licenses of the software used to train the models. So, in this case, it is not merely that divination’s function is obfuscating responsibility; obfuscating responsibility also functions as divination’s profit model.
Here we might also wish to address the role of “black boxing” (Latour 1999). A “black box” refers to the (accepted) opaqueness of some inner workings, an inability to see and foresee internal processes. For algorithms, formalized “black boxing” operates in service of the divinatory ideals of apersonality and “ostensive detachment,” and in the potential sidestepping of accountability. An algorithm’s computational inner workings may be protected explicitly, in the name of competitive advantage or intellectual property. They may be obscured more implicitly behind a rhetoric of processual complexity and deep neural networks. In either instance, the algorithms are effectively closed off to examination, “to political debate, visibility, or accountability” (Campolo and Crawford, 2019, 12). Yet it is the rhetoric around the term, its common usage as a shrug of the shoulders, which enables divination’s liberatory effect.
Anthropological research has shown that divination is rarely concerned with truth. We readily accept and explain away divination’s predictive failures (Hong and Henrich 2021), perhaps for algorithms as statistical outliers or biased training data. Accuracy is not what is at stake in divination. In some analyses, divination is in fact everything else: “emotional reassurance, a tool for restoring and sustaining a social structure, an instrument for making decisions, building consensus, and establishing political legitimacy, or an aid for maintaining a cognitive order” (Myhre 2006, 313; cited in Boyer 2020, 100); everything aside from “an attempt to acquire accurate information about some matter of interest” (Boyer 2020, 100).
Scientific researchers and software engineers may argue that algorithmic practices, which we collate here as divination work, are needed to manage an overabundance of data, or even as “best practice” in the management of contingencies and uncertainty. But scapulimancy does more than guide hunters to game. It moves the cost of uncertainty and the potential for poor outcome away from the shoulders of any individual (Boyer 2020, 109). The result is more than just displacing responsibility. Divination endows the client with the impetus to take action in the face of uncertainty, the authorization to “do something” when the only known is the unknown. Medical researchers used machine learning to predict the development of Covid severity in individual patients, or track its possible spread (Khemasuwan and Colt 2021), under pandemic conditions characterized by a high level of uncertainty and fear. The accuracy of the predictions themselves were not nearly as important as the sociotechnological efforts and pursuit of consensus and even hope in the face of an unprecedented global health crisis.
As with all cultural practices, algorithms present the potential for influence and domination as well as empowerment and agency. Through a lens of divination, we are able to foreground ideas of detachment, neutrality, and apersonality as part of the very imagination and design of algorithmic practices and their “ostensive detachment.” We are better positioned to probe the potential impact and agenda of “black box” rhetoric or the distribution of accountability. We are also able to see the opportunities for social consensus and collaborative action, and even comfort, drawn from these practices. More than a mathematical or mechanical achievement then, algorithms are understood within a rich sociocultural history of divinatory ritual and practice.
Acknowledgements
Thanks go to all the scientists who participated in Rebecca’s research project, which was also supported by the Japan Society for the Promotion of Science’s Grant-in-Aid for Scientific Research (C) 20K01188. We would also like to extend our gratitude to the Code Ethnography Collective (CECO). The concept for this publication first arose from the insightful discussions we had with members of CECO.
Footnotes
[1] We use the word algorithm loosely to gather practices which involve or incorporate the use of various algorithmic technologies including machine and deep learning and other forms of AI. Other scholars have also noted the similarity, even if only rhetorically, between algorithms, magic and divination (cf. Larsson and Viktorelius 2022; Thomas and Veldhuis 2019; Campolo and Crawford 2019; Elish and boyd 2017).
[2] Mechanical divination differs in some respects from other types, as it relies not on a diviner’s direct channeling of spirits, but on the use, manipulation and interpretation of ritual objects.
[3] Even in classical divination, “random” isn’t always entirely random; math and formulae are also present in some relatively ancient divinatory practices (Archer 2002).
[4] EVP stands for electronic voice phenomena.
[5] In November 2022, OpenAI released ChatGPT, a large language model (LLM) with a user interface that was easier to use and required less technical knowledge than anything that had come before. ChatGPT gives good coding advice also, but because of its dialogue-oriented interface, and different training materials, it works better answering more general questions and problem-solving related to software development.
[6] Many contemporary LLM interfaces are so easy that anyone can use them. Unlike the original Greek oracle, one can “Ask Delphi” (Allen institute for AI 2021) directly for an unequivocal ethical judgment. However, perhaps the lack of specialists is due to the novelty of AI “godbots” (cf. Keane & Shapiro 2023); many people are suggesting that a profession of a prompt engineer is emerging, analogous to the operator of a divination system.
[7] Diviners are not always local, however. Boyer details that: “[I]n many societies people travel a long distance to consult a diviner with no connections to their own social environment (Myhre 2006:319; Silva 2014:1179). In the perspective presented here, there is no positive reason why faraway diviners should be more reliable but some reason to expect local ones to be potentially biased and therefore less reliable” (2020, 108).
References
Amelang, Katrin and Susanne Bauer. 2019. “Following the Algorithm: How Epidemiological Risk-Scores Do Accountability.” Social Studies of Science 49(4): 476–502. https://doi.org/10.1177/0306312719862049.
Argyrou, Vassos. 2002. Anthropology and the Will to Meaning: A Postcolonial Critique. London, UK: Pluto Press.
Ascher, Marcia. 2002. Mathematics Elsewhere: An Exploration of Ideas Across Cultures. Princeton, NJ: Princeton University Press.
Aven, Terje. 2013. “On the Meaning of a Black Swan in a Risk Context.” Saf Sci 57:44–51.
Bascom, William. 1969. The Yoruba of Southwestern Nigeria. New York, NY: Holt, Rinehart & Winston.
Boyer, Pascal. 2020. Why Divination?: Evolved Psychology and Strategic Interaction in the Production of Truth. Current Anthropology 61(1): 100-123.
Campolo, Alexander and Kate Crawford. 2019. “Enchanted Determinism: Power without Responsibility in Artificial Intelligence.” Engaging Science, Technology, and Society 6: 1-19. https://doi.org/:10.17351/ests2020.277
Carlson, Rebecca. 2022. “Cells in the “Border Zone”: The Translation of Data in Bioscientific Practice.” RAI Mobilising Methods in Medical Anthropology Conference, January 18-21, 2022.
Du Bois, John W. 1993. Meaning without Intention: Lessons from Divination. In Jane H. Hill and Judith T. Irvine (eds). Responsibility and Evidence in Oral Discourse. Cambridge, UK : Cambridge University Press.
Elish, M.C. and boyd, danah. 2017. “Situating methods in the magic of big data and artificial intelligence.” Communications Monographs 85(1): 57-80. https://doi.org/10.1080/03637751.2017.1375130
Espirito Santo, Diana 2013. “Fluid Divination: Movement, Chaos, and the Generation of “Noise” in Afro-Cuban Spiritist Oracular Production.” Anthropology of Consciousness 24(1): 32–56. https://doi.org/10.1111/anoc.12006
Evans-Pritchard, E.E. 1976 [1937]. Witchcraft, Oracles, and Magic Among the Azande. Oxford, UK: Clarendon Press.
Hong, Ze, & Henrich, Joseph. 2021. “The Cultural Evolution of Epistemic Practices: The Case of Divination.” Human Nature 32(3): 622–651. https://doi.org/10.1007/s12110-021-09408-6
Jasanoff, Sheila and Sang-Hyun Kim. 2015. Dreamscapes of Modernity: Sociotechnical Imaginaries and the Fabrication of Power. Chicago, IL: University of Chicago Press.
Keane, Webb and Scott J. Shapiro 2023. Deus Ex Machina: The Dangers of AI Godbots. The Spectator 29 July. https://www.spectator.co.uk/article/deus-ex-machina-the-dangers-of-ai-godbots/
Khemasuwan, Danai and Henri G. Colt. 2020. “Applications and Challenges of AI-based Algorithms in the COVID-19 Pandemic.” BMJ Innov 7:387–398. https://doi.org/10.1136/bmjinnov-2020-000648
Larsson, Simon and Martin Viktorelius. 2022. “Reducing the Contingency of the World: Magic, Oracles, and Machine-learning Technology.” AI & Soc. https://doi.org/10.1007/s00146-022-01394-2
Latour, Bruno and Steve Woolgar. 1986. Laboratory Life: The Construction of Scientific Facts. Princeton, NJ: Princeton University Press.
Latour, Bruno 1999. Pandora’s Hope: Essays on the Reality of Science Studies. Cambridge, MA: Harvard University Press.
Myhre, Knut Christian. 2006. “Divination and Experience: Explorations of a Chagga Epistemology.” Journal of the Royal Anthropological Institute 12(2): 313–330. https://doi.org/10.1111/j.1467-9655.2006.00293.x
Silva, Sónia. 2014. “Mind, Body and Spirit in Basket Divination: An Integrative Way of Knowing.” Religions 5(4): 1175–1187. https://doi.org/10.3390/rel5041175
Thomas, Matthew Gwynfryn and Djuke Veldhuis. 2019. “Is Artificial Intelligence Magic?: What Does the Anthropology of Magic Reveal About How People Create and Use AI?” Sapiens. https://www.sapiens.org/culture/ai-as-magic/
Werbner, Richard. 1973. “The Superabundance of Understanding: Kalanga Rhetoric and Domestic Divination.” American Anthropologist 75(5): 1414–1440.
4 Comments
Carlson, Wilenius, and Corliss make some nice parallels between divination and algorithms. They use ideas of putting divination (or algorithms) in black boxes, making them opaque. In not unrelated work I’ve played with Susan Leigh Star’s idea of boundary objects to capture the way that the clients of divination may not know about the details of the divinatory system they are consulting, and indeed may not share ontologies with the diviner. I use this to shift the emphasis from the diviners to their clients, and to ask somewhat different questions – that are relevant here – what do clients do with the results of divination? If we view it as ‘advice’ then what do people do with that advice? Sometimes it helps focus the mind, helps clarify what we want to do even if we do not follow the advice being given!
Applied to algorithms and contemporary high tech worlds it nicely shifts to how computer systems are used to mediate social relations and how many customers are disempowered / affected in the process. It is more about politics and sociology than about technology. Therefore messier. Little wonder many prefer to talk about the technology. As anthropologists we should resist this.
See
Star, Susan Leigh 2010. “This is Not a Boundary Object: Reflections on the Origin of a Concept.” Science, Technology, & Human Values 35 (5):601-17. doi: 10.1177/0162243910377624.
Zeitlyn, David
2020. Mambila Divination: Framing Questions, Constructing Answers (Routledge Studies in Anthropology). London: Routledge. HB: ISBN 9780367199500. PB: ISBN 9781032174082
‘Divination and ontologies: a reflection’ 2021 Social Analysis 65(2), 139-160. 139–160 doi:10.3167/sa.2021.650208 Online ISSN: 1558-5727 Print ISSN: 0155-977X https://www.berghahnjournals.com/view/journals/social-analysis/65/2/sa650208.xml
David, Thanks so much for sharing your research! You get on to great (messy) stuff in your reply. I like your use of Star in this case.
Looking forward to reading your articles,
Rebecca
David is absolutely correct to point out that, as anthropologists, we should not focus our analytic gaze only on the technology when we consider the parallels between mechanical divination and contemporary computerized algorithms. At the same time, there are some specific ethnographic contexts in which it would be incorrect to argue, as David does, that “it is more about politics and sociology than about technology.” For example, one of my ongoing research projects has focused on artists in different artistic domains who design and use art-producing computerized algorithms and technologies as creative partners that are meant to broaden those artists’ creative horizons—a practice that I have analyzed in terms of mechanical divination (in addition to other frameworks). In such cases, the “clients” of the divinatory systems are also the people who design those systems, and thus the technology intimately shapes and is shaped by politics and sociology. It is impossible to analyze the one without the other.
See:
Wilf, Eitan
2023 The Inspiration Machine: Computational Creativity in Poetry and Jazz. Chicago: University of Chicago Press.
2013. “Sociable Robots, Jazz Music, and Divination: Contingency as a Cultural Resource for Negotiating Problems of Intentionality,” American Ethnologist 40(4):605-618.
Thanks for your comment Eitan – I’m also interested in cases, where people’s interaction with these kinds of divinatory systems involve a problematization and a possible reworking of the technology that they interact with, reconfiguring genres previously understood to belong exclusively to humans.
Looking forward to read your work on this! (BTW, Ilana Gershon discusses your new book in an essay recently published in Suomen Antropologi: https://journal.fi/suomenantropologi/article/view/137824)
1 Trackback