Editor’s Note: This is the fourth post in our Law in Computation series.
War is an experiment in catastrophe; yet, policymakers today believe chance can be tamed and ‘ethical war’ waged by simply increasing battlefield technology, systematically removing human error/bias. How does an increasingly artificially intelligent battlefield reshape our capacity to think ethically in warfare (Schwartz, 2016)? Traditional ethics of war bases the justness of how one fights in war on the two principles of jus in bello (justice in fighting war) ethics: discrimination and proportionality, weighted against military necessity. Although how these categories apply in various wars has always been contested, the core assumption is that these categories are designed to be an ethics of practical judgment (see Brown, 2010) for decision-makers to weigh potentially grave consequences of civilian casualties against overall war aims. However, the algorithmic construction of terrorists has radically shifted the idea of who qualifies as a combatant in warfare. What then are the ethical implications for researchers and practitioners for a computational ethics of war?
The drive of US policy-makers to prevent terrorist attacks before they happen has led to a global drone campaign whereby mere suspicion has been promoted to the rank of a scientific calculus of probabilities upon which an instantaneous death sentence is executed. This post, as a snapshot of my dissertation research, looks at the machine-learning technology that has already been deployed in war, where this technology will likely be in the next few years, and some implications for how we think about war ethics today.
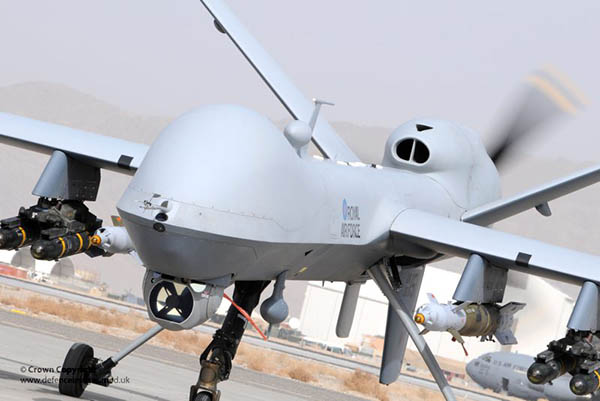
A Reaper MQ-9 Remotely Piloted Air System (RPAS). Photo:Defence Images (Creative Commons).
SKYNET, Big Data, and Death by Drone
SKYNET was the joint NSA and CIA operation over Yemen and Pakistan where the NSA gathered mobile phone SIM card metadata upon which to base “targeted” drone strikes in Yemen, Pakistan, and Somalia, and was of hotly contested legality. The term “targeted” in targeted killing was misleading as it implied that the US knew who it was striking instead of what it was striking with Hellfire missiles from the Predator drones loitering above. In fact, what it was striking was a statistical probability: “patterns of life” (the NSA’s term) based upon heterogeneous correlations to construct a probability of ‘terroristness’ (my terminology) A leaked NSA PowerPoint detailed the secret assassination campaign, which used cloud-based behavior analytics to determine a target’s probability of ‘terroristness’ to receive a Hellfire missile via Predator drone from above. It is no secret that this has become the underpinning logic of the CIA drone campaign: as General Michael Hayden (former director of the NSA and CIA) bluntly stated, “We kill people based on metadata.” However, as the PowerPoint noted, the “highest scoring selector” based on “pattern of life, social network, and travel behavior” metadata—that is, the target most likely to exhibit ‘terroristness’—turned out to be Ahmad Muaffaq Zaidan, Al Jazeera’s longtime Islamabad bureau chief.
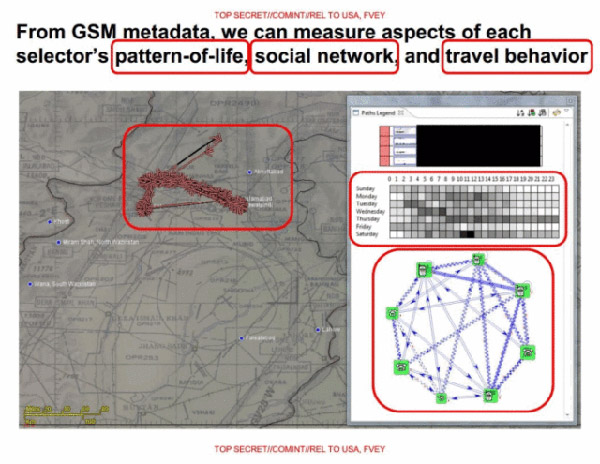
The NSA Powerpoint. Photo: The Intercept.
SKYNET works like a typical modern Big Data business application. The program collects metadata and stores it on NSA cloud servers, extracts relevant information, and then applies machine learning to identify leads for a targeted campaign. However, instead of trying to sell the targets something like the business applications, this campaign executes the CIA/NSA “Find-Fix-Finish” strategy using Hellfire missiles to take out their target. In addition to processing logged cellular phone call data (so-called “DNR” or Dialed Number Recognition data, such as time, duration, who called whom, etc.), SKYNET also collects user location, allowing for the creation of detailed travel profiles. The turning off a mobile phone gets flagged as an attempt to evade mass surveillance. Users who swap SIM cards, naively believing this will prevent tracking, also get flagged (the ESN/MEID/IMEI burned into the handset makes the phone trackable across multiple SIM cards). Given the complete set of metadata, SKYNET pieces together people’s typical daily routines—who travels together, has shared contacts, stays overnight with friends, visits other countries, or moves permanently.
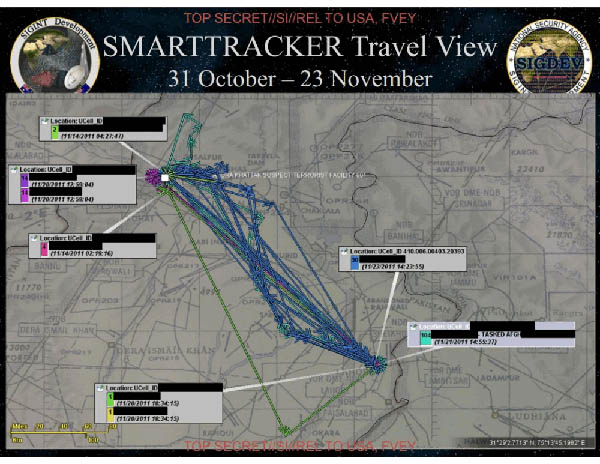
SKYNET Tracking Map. Photo: The Intercept.
The slides indicate, overall, that the NSA machine-learning algorithm uses more than 80 different properties to rate people on their ‘terroristness.’ Hence, the idea of who qualifies as a combatant and becomes a legitimate target – in line with jus in bello ethics – has been eroded. The ethical implications are staggering as the subjectivity of the combatant has been replaced by the process of data construction, which undercuts the rationale for why it is ethically permissible to kill a combatant in war—one’s subjectivity. Ultimately, ethical practical judgment has been replaced by computation, rendering complex ethico-political dilemmas of warfare knowable, quantifiable, objective, and neutral.
Autonomous Weapons, Killer Robots, and the Future or War
We know now that programmer biases are always written into the code. Thus, human bias has not been eliminated, but shifted, from practical judgment to computation, which presents its own practical and ethical quandaries. In April 2017, the Dept. of Defense established its Algorithmic Warfare Cross-Functional Team (Project Maven), whose AI is already “hunting terrorists” in Syria and Iraq. AI for drone video deployed in Syria thus far has simply analyzed/characterized objects to aid in the avalanche of data. Although the machine learning algorithm was trained on thousands of hours of drone video feed, ever-changing environments of warzones make it error-prone; thus, even as it “is trained to identify people, vehicles and installations” it still “occasionally mischaracterizes an object.” Thus, autonomous weapons have not been fully deployed, but the campaign to stop killer robots has been gaining traction. Elon Musk and others have urged a preemptive UN ban on autonomous weaponry.
There are both contingent and intrinsic arguments against killer robots for executing and generating targeting decisions (Leveringhaus, 2016). The contingent argument correctly states that the technology is currently not advanced enough to autonomously target on the battlefield. Executing a targeting decision is simply an artificially intelligent drone deciding between targets already deemed legitimate by the programmer by applying the jus in bello criteria before deployment. However, generating a targeting decision, the robot must apply the criteria and assess whether a human is a legitimate target or not (discrimination) and a calculation of whether a particular course of action is likely to cause excessive harm to those who cannot be intentionally targeted (proportionality). The problem is such that killer robots will find it hard to determine “what constitutes proportionate and necessary harm,” as the application of the jus in bello criteria are highly context dependent (Leveringhaus, 2016: 5).
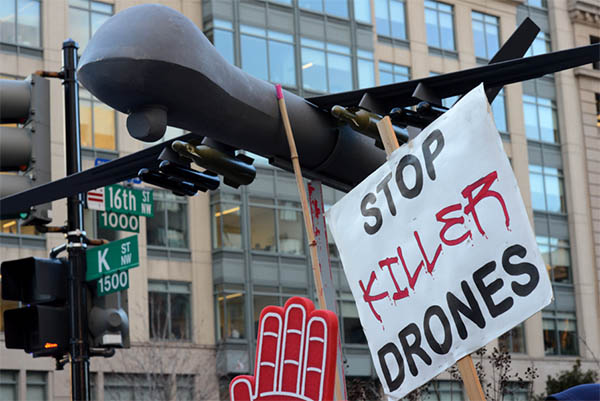
Drone Protest. Photo:Stephen Melkisethian (Creative Commons).
Techno-Ethics, AI, and the Act of Killing
Many social scientists today believe the nature of warfare is timeless and fixed, and past theorists such as Thucydides and Clausewitz put their fingers on these universal truths. However, I and many other just war ethicists believe that the search for transcendent ethical principles in war is folly, and a more case-based understanding of the concrete circumstances of dilemmas in warfare a more fruitful endeavor (see: Walzer, 1977; Jonson & Toulmin, 1990; Toulmin, 1992; Brown, 2010). Thus, the idea that moral judgment in the complex on-the-ground ever-evolving circumstances in war is simply an enduring illusion, with AI being its most technologically advanced iteration to date.
In the end, complex ethico-political dilemmas in warfare cannot be solved via algorithmic calculation. War-makers have attempted to replace an ethics of practical judgment with a computational machine-learning ethics of war. However, the intrinsic argument against killer robots remains: there is no general rule for the proportionality criterion where human lives are ‘weighed’ and ‘balanced’ by AI. Robots lack agency–the ability to do otherwise; thus, by eliminating the negative aspects of human agency (bias, panic, dehumanization, error, etc.) machines also eliminate the positive human elements (empathy, pity, compassion, etc.)–i.e. the decision not to pull the trigger even when you may be legally justified in doing so (Leveringhaus 2016: 15).
One cannot program an ethics of practical judgment into autonomous machines of warfare, nor can Big Data be the basis upon which drone assassinations are justified. Techno-logical solutions to complex dilemmas of war cannot bring to fruition the Enlightenment ideals of a rational Cartesian man, it can only remove us one step further from the act of killing; an ultimately futile attempt to escape the human condition.
The state of play in AI and contemporary warfare vis-à-vis war ethics has been the focus of my contribution to the UC Irvine Technology, Law & Society (TLS) interdisciplinary working group. These issues have been raised in the tech industry as a handful of CEOs have called for hiring humanities majors to help think through broader social consequences that a purely technical mindset may miss. This TLS group has allowed me to put forth bold questions about how these AI and algorithmic technologies reshape how we think about ethics; and my hunch thus far is that killer robots will be here shortly, and that computational judgment can never fully encompass the practical judgment and human agency of the act of killing.
References
Brown, Chris
2010 Practical Judgement in International Political Theory: Selected Essays. New York: Routledge.
Jonson, Albert R. and Stephen Toulmin
1990 The Abuse of Casuistry: A History of Moral Reasoning. Berkeley: University of California Press.
Leveringhaus, Alex
2016 “What’s So Bad About Killer Robots?” Journal of Applied Philosophy (Online Issue Available at): http://dx.doi.org/10.1111/japp.12200.
Schwarz, Elke
2016 Prescription Drones: On the Techno-Biopolitical Regimes of Contemporary ‘Ethical Killing.’ Security Dialogue 47 (1): 59-75.
Toulmin, Stephen
1992 Cosmopolis: The Hidden Agenda of Modernity. Chicago: The University of Chicago Press.
Walzer, Michael
1977 Just and Unjust Wars: A Moral Argument with Historical Illustrations. New York: Basic Books.
1 Comment
“However, the intrinsic argument against killer robots remains: there is no general rule for the proportionality criterion where human lives are ‘weighed’ and ‘balanced’ by AI”
I’m as much against killer robots as any other puny human underling. But I am not sure I buy the argument that weapons in the hands of human subjectivity is any better. Police shoot more black citizens than white; they too say that “human lives are weighted and balanced”. If Black Lives Matter wants to build an in-ear AI assistant that helps cops make better decisions, I’m all for it.
1 Trackback