Amid global climate impacts, vulnerable communities—including indigenous peoples, farmers, fisherfolk, and low-income groups—are frequently expected to adapt, change, and build resilience to uncertain climatic futures. Under these changing conditions, what knowledge practices and frameworks should guide the decision-making of vulnerable communities in addressing climate challenges? What knowledge sources and perspectives should be considered when developing resilience policies and plans, from the supranational to the local level?
Recent advances in the field of Artificial Intelligence (AI) have generated a wave of enthusiasm amongst various stakeholders to adopt and deploy AI solutions to tackle climate change. Recently, the UNFCCC Technology Mechanism launched a five-year work plan (2023-27) to explore the role of “AI as a powerful technological tool…in advancing and scaling up transformative climate solutions, particularly for the most vulnerable communities” (UNFCCC 2023). Similarly, UNESCO along with UNDP, and other organisations have formed the “AI for Planet ” alliance with the aim to “drive AI solutions for climate change at scale” (UNESCO, 2022). Governments, private corporations, and start-ups are increasingly embracing the idea of AI as a transformative tool for climate action. Examples include Microsoft’s AI for Earth initiative (Spencer 2018), which supports biodiversity conservation and agriculture sustainability projects at various locales across the globe. ‘Mapping the Andean Amazon Project’ (MAAP), an international nonprofit, uses remote sensing to track deforestation and forest fires in the Amazon in real-time (Finer et al. 2018). In India, the Ministry of Earth Sciences’ ACROSS program (Ikigai Law 2023) has plans to deploy AI to build weather models and visualization systems and to create more accurate weather and natural disaster forecasting systems. While AI solutions for climate change span a wide range of applications, from climate modeling to precision agriculture to the development of early warning systems, the extent to which such technologies will deliver on their promise is far from clear. In fact, the use of AI-based solutions for climate change could give rise to novel and systemic risks.
The use of AI-based knowledge systems for climate adaptation and resilience needs careful consideration as these are often geared towards addressing adaptation responses of historically marginalized and vulnerable communities. Such systems often operate in contexts underlined by unequal power relations and implicit biases (Crawford 2021).The channelling of adaptation strategies through AI-based solution engineering poses the risk of perpetuating what Miranda Fricker calls epistemic injustice (2007). Fricker outlines the concept of epistemic injustice as an injustice that is “done to someone specifically in their capacity as a knower” (2007). It highlights the unfair discrimination faced by individuals whose lives hinge on knowledge and scientific understanding through a systematic devaluation, marginalization, or exclusion of certain forms of knowledge. Epistemic injustices can arise when AI-based solutions prioritize certain types of knowledge, such as scientific or quantitative data, while undervaluing or excluding other forms of knowledge, including indigenous, local, or traditional knowledge systems. Fricker identifies two forms of epistemic injustice. The first is testimonial injustice, when a person’s testimony is given less credibility or deemed less reliable based on prejudiced assumptions about their social identity, such as race, gender, or socioeconomic status. Testimonial injustice can lead to the silencing or marginalization of certain voices, as their knowledge and experiences are devalued or dismissed. The second form is hermeneutic injustice, which arises when individuals cannot fully understand or make sense of their own experiences due to a lack of shared conceptual resources. This can occur when dominant narratives or cultural norms exclude or misrepresent certain groups, preventing them from articulating their own perspectives and shaping the collective understanding of important issues. In the case of science, the high degree of cognitive authority placed on its institutions exacerbates the epistemic injustices already at play.
Scientific knowledge and knowledge practices are never neutral and can play a role in perpetuating injustices. The production and dissemination of scientific knowledge is often shaped by the interests, perspectives, and values of those who hold the power to define research agendas and interpret findings. Climate science, too, is emblematic of the underlying power structures, cultural biases, and historical contexts that shape epistemic authority. Climate action, often manifested in the form of highly engineered or scientifically informed policies and projects, frequently functions to the detriment of vulnerable groups and communities. For instance, consider the unfolding of projects where urban risk mapping and management projects (Mendes Barbosa and Walker 2020) disproportionately led to the clearance of favelas (slums) in Brazil. Or, where science-backed government policies work directly against the human rights of indigenous communities (Tsosie 2012). Socio-technical imaginaries of resilience tend to hide complex power dynamics (Yarina 2018). When adaptation and resilience, involving vulnerable communities, are conceptualized, and pursued through specific socio-technical imaginaries, it is important to examine who has the power to shape these narratives and how power is distributed within them.
The introduction of AI-based technological solutions in such a scenario can further accentuate the issue due to several reasons. First, AI applications produce an over-reliance on computable and large-scale datasets, which provide a foundation for algorithmic reasoning, to which traditional and local knowledge need not necessarily map onto. These datasets encompass diverse sources of environmental data, including satellite imagery, weather observations, carbon emissions data, etc., within which subjective and contextual knowledge features very little.
Second, epistemic injustices of the nature of testimonial injustice can also evolve into hermeneutic injustices (Byskov 2021), when there is systemic exclusion against a knower’s experiences and knowledge from the collective pool of knowledge (Sardelli 2022). In the context of AI, it can be argued that hermeneutic injustice is written into how AI systems are built and operated. The black-box problem is an intrinsic aspect of AI that leads to opacity and an inability to demystify the decision-making processes of most machine and deep learning applications of AI. The complexity and opacity of these technologies restricts the capacity to challenge, understand, or question to an elite few while excluding others who are either participating or being made subject to its outcomes (Symons and Alvarado 2022). Epistemic injustices can also be perpetuated when decision-making processes and policy recommendations derived from AI systems are presented as objective and unbiased, despite being influenced by underlying assumptions and biases.
Third, the attraction towards adopting AI-based technological solutions lies in their ability to be scalable and replicable (Hanna and Park 2020). Tools developed with the financial resources, computational and technological capacities, and data/contexts of the Global North are often transposed to combat climate-related crises in developing countries with widely divergent contexts. However, in such cases, adaptation and resilience strategies offer unique challenges to AI, as adaptation is inherently localized, heterogeneous, and contextual. Different communities adapt differently and possess different ideas of what constitutes resilience.
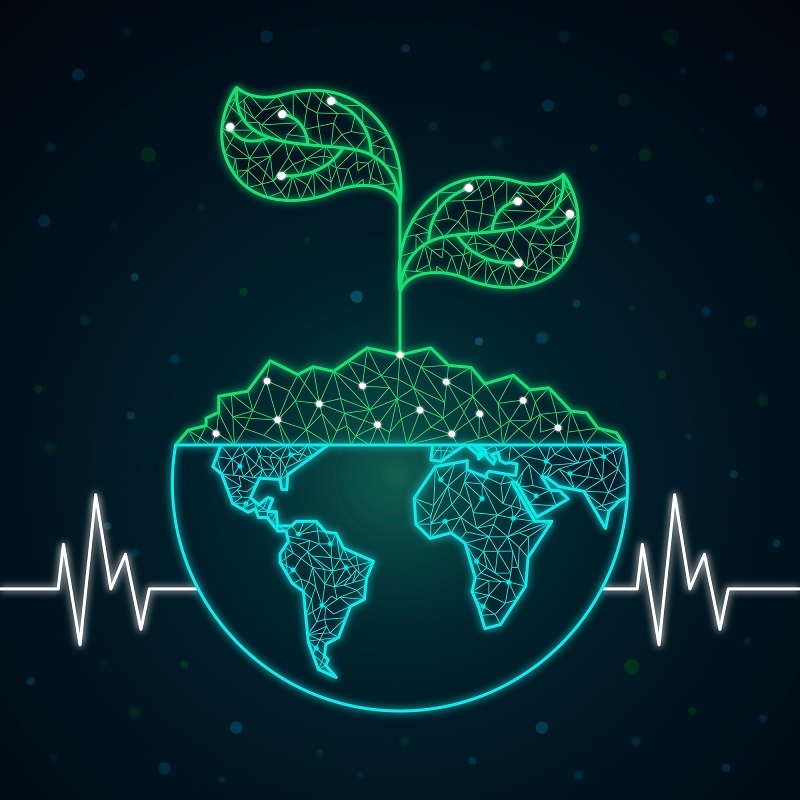
Image by Freepik
For instance, take the case of ViEWS, which is a “machine learning based tool designed to forecast the probability of violence at a country and sub-national level, 36 months into the future” (Ballesteros Figueroa 2022). Produced in Sweden, the tool has been used to provide “multiple types of violence forecasts in Africa: state based, non-state, one-sided, drought-driven conflicts, and migrations.” The core issue with such tools, Ferguaro notes, is not only the risks of inaccurate predictions, but also the risks of undermining the ability and provision of support to affected communities in evaluating their risks independently, thereby creating a dependency on devices such as these. Further, inaccuracies can also lead to misguided policy decisions and ineffective allocation of resources, undermining the overall effectiveness of climate action. A similar problem arises in the case of the introduction of precision agricultural technologies in the pursuit of climate-smart agriculture in India. SA Malik argues that the promotion of techniques, tips, and incentives to farmers to adopt specific agricultural practices (Malik 2022), in combination with neoliberal regimes of contract farming and datafied agricultural insurance schemes, not only deskills and robs farmers of their agency, but also makes them vulnerable to debt relations, and dispossession.
Exclusionary biases and epistemic injustices, which are symptomatic of several AI-based technologies (Sardelli 2022), can result in a limited understanding of complex environmental issues and hinder the development of comprehensive and contextually relevant climate solutions. Many vulnerable communities that often possess invaluable knowledge about their local ecosystems, weather patterns, and sustainable practices may find their knowledge and perspectives marginalized or dismissed by AI systems that prioritize dominant forms of knowledge.
What solutions must we then seek to counter the perpetuation of epistemic injustices in the context of the use of AI for climate change? There is a need to recognize the epistemic injustices prevalent in AI-based climate solutions and to address them through participatory modalities of climate action. Focusing solely on scalable tech risks overlooking critical underlying issues that are deeply interconnected with environmental challenges. By narrowing our attention to technological solutions alone, we may fail to address the systemic complexities contributing to climate change and environmental degradation. While these technologies may carry real potential, the rush towards scalable, one-size fits all, replicable solutions can come at the cost of ignoring locally-led, traditionally rooted systems of adaptation and resilience.
Acknowledging the cultural, relational, and contextual dimensions of climate change is crucial in the realms of science, policy, and practice. While the deployment of AI has the potential to aid decision-making processes, it must be approached with caution to prevent exacerbating underlying injustices. Human experiences should not be obscured or overlooked in favour of algorithmic solutions.
Grove-White highlights the importance of understanding the significance of contextuality and depth of human values in shaping knowledge and action (Grove-White and Szerszynski 1992). This perspective calls for a human-centred approach that considers the diverse perspectives, experiences, and values of individuals and communities affected by climate change. Moreover, the concept of humility of expertise also urges practitioners to approach their work with empathy and a deep understanding of people’s lived experiences. Iris Murdoch describes this approach as the ability to enter into the lived and felt realities of others with a “patient eye of love” (Murdoch 2013), recognizing its importance in framing effective responses to complex challenges.
Humanizing science, policy, and practice can create spaces that value and integrate diverse knowledge systems, foster meaningful engagement with affected communities, and promote inclusive decision-making processes. This approach recognizes the limitations of AI and emphasizes the critical role of human judgment, empathy, and ethical considerations in ensuring responsible and equitable outcomes in addressing climate change and other societal challenges.
References
Ballesteros Figueroa, Jose Antonio. 2022. “Datafication without Participation: When Global North Forecasting Devices Measure Global South Subjects.” The Sociological Review Magazine, July. https://doi.org/10.51428/tsr.exor5409.
Byskov, Morten Fibieger. 2021. “What Makes Epistemic Injustice an ‘Injustice’?” Journal of Social Philosophy 52 (1): 114–31. https://doi.org/10.1111/josp.12348.
Crawford, Kate. 2021. Atlas of AI: Power, Politics, and the Planetary Costs of Artificial Intelligence. New Haven London: Yale University Press.
Finer, Matt, Sidney Novoa, Mikaela J. Weisse, Rachael Petersen, Joseph Mascaro, Tamia Souto, Forest Stearns, and Raúl García Martinez. 2018. “Combating Deforestation: From Satellite to Intervention.” Science 360 (6395): 1303–5. https://doi.org/10.1126/science.aat1203.
Fricker, Miranda. 2007. Epistemic Injustice. Oxford University Press. https://doi.org/10.1093/acprof:oso/9780198237907.001.0001.
Grove-White, Robin, and Bronislaw Szerszynski. 1992. “Getting Behind Environmental Ethics.” Environmental Values 1 (4): 285–96. https://doi.org/10.3197/096327192776680016.
Hanna, Alex, and Tina M. Park. 2020. “Against Scale: Provocations and Resistances to Scale Thinking.” https://doi.org/10.48550/ARXIV.2010.08850.
Ikigai Law. 2023. “Landscaping the Use of AI for Climate Change: Addressing Challenges and Risks,” 2023. https://www.ikigailaw.com/landscaping-the-use-of-ai-for-climate-change-addressing-challenges-and-risks/#acceptLicense.
Malik, S. Ali. 2022. “Linking Climate-Smart Agriculture to Farming as a Service: Mapping an Emergent Paradigm of Datafied Dispossession in India.” The Journal of Peasant Studies, November, 1–23. https://doi.org/10.1080/03066150.2022.2138751.
Mendes Barbosa, Luciana, and Gordon Walker. 2020. “Epistemic Injustice, Risk Mapping and Climatic Events: Analysing Epistemic Resistance in the Context of Favela Removal in Rio de Janeiro.” Geographica Helvetica 75 (4): 381–91. https://doi.org/10.5194/gh-75-381-2020.
Murdoch, Iris. 2013. The Sovereignty of Good. Routledge.
Sardelli, Martina. 2022. “Epistemic Injustice in the Age of AI.” Aporia 22: 44–53.
Spencer, Geoff. 2018. “AI for Earth: Helping Save the Planet with Data Science.” Microsoft Asia, 2018. https://news.microsoft.com/apac/features/ai-for-earth-helping-save-the-planet-with-data-science/.
Symons, John, and Ramón Alvarado. 2022. “Epistemic Injustice and Data Science Technologies.” Synthese 200 (2): 87. https://doi.org/10.1007/s11229-022-03631-z.
Tsosie, Rebecca. 2012. “Indigenous Peoples and Epistemic Injustice: Science, Ethics, and Human Rights.” Wash. L. Rev. 87: 1133.
UNESCO. 2021. “AI for the Planet: Highlighting AI Innovations to Accelerate Impact,” 2021. https://www.unesco.org/en/articles/ai-planet-highlighting-ai-innovations-accelerate-impact.
1 Trackback